The U-Shape of Automation
As we look ahead, we see these three trends not only accelerating but also evolving. For instance, new research by David Autor and David Dorn has put an interesting twist on the SBTC story. They find that the relationship between skills and wages has recently become U-shaped. In the most recent decade, demand has fallen most for those in the middle of the skill distribution. The highest-skilled workers have done well, but interestingly those with the lowest skills have suffered less than those with average skills, reflecting a polarization of labor demand.
This reflects an interesting fact about automation. It can be easier to automate the work of a bookkeeper, bank teller, or semi-skilled factory worker than a gardener, hairdresser, or home health aide. In particular, over the past 25 years, physical activities that require a degree of physical coordination and sensory perception have proven more resistant to automation than basic information processing, a phenomenon known as Moravec’s Paradox’. For instance, many types of clerical work have been automated, and millions of people interact with robot bank tellers and airport ticket agents each day. More recently, call center work—which was widely offshored to India, the Philippines, or other low-wage nations in the 1990s—has increasingly been replaced by automated voice response systems that can recognize an increasingly large domain-specific vocabulary and even complete sentences.
In contrast, vision, fine motor skills, and locomotion have been much harder to automate. The human brain can draw on highly specialized neural circuitry, refined by millions of years of evolution, to recognize faces, manipulate objects, and walk through unstructured environments. Although multiplying five-digit numbers is an unnatural and difficult skill for the human mind to master, the visual cortex routinely does far more complex mathematics each time it detects an edge or uses parallax to locate an object in space. Machine computation has surpassed humans in the first task but not yet in the second one.
Notes:
People in semi-skilled jobs have been the ones most automated out of jobs, while highly-technical and more menial jobs have remained.
Folksonomies: employment automation
Taxonomies:
/business and industrial/business operations/management/business process (0.414205)
/technology and computing (0.218415)
/business and industrial (0.196315)
Keywords:
semi-skilled factory worker (0.902678 (neutral:0.000000)), home health aide (0.849056 (positive:0.436603)), robot bank tellers (0.835011 (neutral:0.000000)), basic information processing (0.834575 (positive:0.361297)), large domain-specific vocabulary (0.833471 (neutral:0.000000)), airport ticket agents (0.828814 (neutral:0.000000)), fine motor skills (0.827154 (positive:0.350235)), automated voice response (0.826651 (neutral:0.000000)), semi-skilled jobs (0.789632 (neutral:0.000000)), David Autor (0.726980 (positive:0.545313)), Automation People (0.716852 (neutral:0.000000)), menial jobs (0.716403 (negative:-0.436405)), David Dorn (0.710894 (positive:0.545313)), lowest skills (0.708979 (neutral:0.000000)), skill distribution (0.699128 (negative:-0.334544)), interesting twist (0.698961 (positive:0.545313)), labor demand (0.698950 (negative:-0.288654)), new research (0.697760 (positive:0.545313)), average skills (0.697707 (neutral:0.000000)), SBTC story (0.696928 (positive:0.545313)), recent decade (0.695626 (neutral:0.000000)), interesting fact (0.693901 (positive:0.839682)), highest-skilled workers (0.690870 (neutral:0.000000)), low-wage nations (0.688853 (neutral:0.000000)), visual cortex (0.687045 (neutral:0.000000)), clerical work (0.686346 (neutral:0.000000)), sensory perception (0.685399 (positive:0.361297)), difficult skill (0.682693 (negative:-0.223787)), unstructured environments (0.682358 (neutral:0.000000)), physical activities (0.682276 (positive:0.361297))
Entities:
Automation People:Organization (0.938606 (neutral:0.000000)), David Autor:Person (0.620587 (positive:0.545313)), David Dorn:Person (0.553488 (positive:0.545313)), SBTC:Organization (0.544442 (positive:0.545313)), India:Country (0.459992 (neutral:0.000000)), Philippines:Country (0.440389 (neutral:0.000000)), five-digit:Quantity (0.440389 (neutral:0.000000)), 25 years:Quantity (0.440389 (neutral:0.000000))
Concepts:
Brain (0.965876): dbpedia | freebase | opencyc
Motor control (0.831672): dbpedia | freebase
Mind (0.794289): dbpedia | freebase | opencyc
Automation (0.709719): dbpedia | freebase
Human brain (0.656155): dbpedia | freebase
Psychology (0.653100): dbpedia | freebase | opencyc
Automated teller machine (0.625667): dbpedia | freebase | opencyc
Perception (0.624533): dbpedia | freebase | opencyc
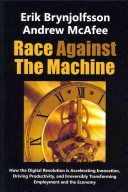
