The Problem of Machine-Aggregated Knowledge
he nuts and bolts of artificial-intelligence research can often be more usefully interpreted without the concept of AI at all. For example, in 2011, IBM scientists unveiled a “question answering” machine that is designed to play the TV quiz show Jeopardy. Suppose IBM had dispensed with the theatrics, and declared it had done Google one better and come up with a new phrase-based search engine. This framing of exactly the same technology would have gained IBM’s team as much (deserved) recognition as the claim of an artificial intelligence, but it would also have educated the public about how such a technology might actually be used most effectively.
AI technologies typically operate on a variation of the process described earlier that accomplishes translations between languages. While innovation in algorithms is vital, it is just as vital to feed algorithms with “big data” gathered from ordinary people. The supposedly artificially intelligent result can be understood as a mash-up of what real people did before. People have answered a lot of questions before, and a multitude of these answers are gathered up by the algorithms and regurgitated by the program. This in no way denigrates it or proposes it isn’t useful. It is not, however, supernatural. The real people from whom the initial answers were gathered deserve to be paid for each new answer given by the machine.
Consider too the act of scanning a book into digital form. The historian George Dyson has written that a Google engineer once said to him: “We are not scanning all those books to be read by people. We are scanning them to be read by an AI.” While we have yet to see how Google’s book scanning will play out, a machine-centric vision of the project might encourage software that treats books as grist for the mill, decontextualized snippets in one big database, rather than separate expressions from individual writers. In this approach, the contents of books would be atomized into bits of information to be aggregated, and the authors themselves, the feeling of their voices, their differing perspectives, would be lost. Needless to say, this approach would hide its tracks so that it would be hard to send a nanopayment to an author who had been aggregated.
Notes:
If an AI works by aggregating the works of the sum total of human knowledge, should the humans that discovered that knowledge be compensated? Science works the same way, but ideas remain free.
Folksonomies: creative commons artificial intelligence knowledge automation ai creative works
Taxonomies:
/technology and computing/computer certification (0.446845)
/technology and computing (0.444097)
/art and entertainment/books and literature (0.429652)
Keywords:
phrase-based search engine (0.970192 (positive:0.299893)), artificially intelligent result (0.928189 (positive:0.316664)), real people (0.915507 (positive:0.072655)), historian George Dyson (0.903052 (positive:0.220948)), artificial-intelligence research (0.785524 (negative:-0.452248)), AI works (0.784251 (positive:0.266558)), sum total (0.771477 (positive:0.266558)), Machine-Aggregated Knowledge (0.760171 (positive:0.266558)), human knowledge (0.759263 (positive:0.266558)), AI technologies (0.757913 (neutral:0.000000)), quiz show Jeopardy (0.755501 (negative:-0.238397)), IBM scientists (0.740560 (neutral:0.000000)), question answering (0.739130 (neutral:0.000000)), artificial intelligence (0.739044 (neutral:0.000000)), book scanning (0.734159 (positive:0.434573)), ordinary people (0.733540 (negative:-0.252008)), big data (0.726908 (neutral:0.000000)), Google engineer (0.722280 (positive:0.220948)), digital form (0.720908 (positive:0.352742)), initial answers (0.714610 (negative:-0.244009)), machine-centric vision (0.712607 (positive:0.567059)), individual writers (0.711042 (neutral:0.000000)), new answer (0.710182 (negative:-0.244009)), big database (0.706057 (neutral:0.000000)), separate expressions (0.705692 (neutral:0.000000)), algorithms (0.624216 (negative:-0.027721)), books (0.596134 (positive:0.359533)), way (0.587965 (neutral:0.000000)), technology (0.573112 (positive:0.370169)), approach (0.568902 (negative:-0.431264))
Entities:
IBM:Company (0.980013 (negative:-0.033879)), Google:Company (0.874004 (positive:0.318471)), AI:Organization (0.746542 (positive:0.266558)), AI technologies:Company (0.660994 (neutral:0.000000)), George Dyson:Person (0.617881 (positive:0.220948)), artificial intelligence:FieldTerminology (0.479591 (neutral:0.000000)), search engine:FieldTerminology (0.453922 (positive:0.299893)), engineer:JobTitle (0.430156 (positive:0.220948))
Concepts:
Artificial intelligence (0.968058): dbpedia | freebase | opencyc
Knowledge (0.641852): dbpedia | freebase
Logic (0.605140): dbpedia | freebase | opencyc
Machine learning (0.558441): dbpedia | freebase | opencyc
Computer (0.536540): dbpedia | freebase | opencyc
Scientific method (0.534180): dbpedia | freebase
Alan Turing (0.528908): dbpedia | freebase | opencyc | yago
Natural language processing (0.520925): dbpedia | freebase | opencyc
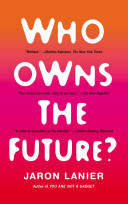
