How IBM's Watson Pattern-Matches to Answer Trivia
The way Watson plays the game also requires massive amounts of pattern matching. The supercomputer has been loaded with hundreds of millions of unconnected digital documents, including encyclopedias and other reference works, newspaper stories, and the Bible. When it receives a question, it immediately goes to work to figure out what is being asked (using algorithms that specialize in complex communication), then starts querying all these documents to find and match patterns in search of the answer. Watson works with astonishing thoroughness and speed, as IBM research manager Eric Brown explained in an interview:
We start with a single clue, we analyze the clue, and then we go through a candidate generation phase, which actually runs several different primary searches, which each produce on the order of 50 search results. Then, each search result can produce several candidate answers, and so by the time we’ve generated all of our candidate answers, we might have three to five hundred candidate answers for the clue.
Now, all of these candidate answers can be processed independently and in parallel, so now they fan out to answer-scoring analytics [that] score the answers. Then, we run additional searches for the answers to gather more evidence, and then run deep analytics on each piece of evidence, so each candidate answer might go and generate 20 pieces of evidence to support that answer.
Now, all of this evidence can be analyzed independently and in parallel, so that fans out again. Now you have evidence being deeply analyzed … and then all of these analytics produce scores that ultimately get merged together, using a machine-learning framework to weight the scores and produce a final ranked order for the candidate answers, as well as a final confidence in them. Then, that’s what comes out in the end.
What comes out in the end is so fast and accurate that even the best human Jeopardy! players simply can’t keep up. In February of 2011, Watson played in a televised tournament against the two most accomplished human contestants in the show’s history. After two rounds of the game shown over three days, the computer finished with more than three times as much money as its closest flesh-and-blood competitor. One of these competitors, Ken Jennings, acknowledged that digital technologies had taken over the game of Jeopardy! Underneath his written response to the tournament’s last question, he added, “I for one welcome our new computer overlords.”
Notes:
And how it does it so fast.
Folksonomies: automation pattern recognition
Taxonomies:
/technology and computing/hardware/computer (0.547177)
/food and drink/food/fast food (0.433029)
/technology and computing (0.404904)
Keywords:
candidate answers (0.925348 (positive:0.341755)), candidate generation phase (0.787982 (positive:0.353751)), unconnected digital documents (0.729621 (negative:-0.461597)), research manager Eric (0.718582 (neutral:0.000000)), different primary searches (0.714028 (neutral:0.000000)), closest flesh-and-blood competitor (0.706838 (neutral:0.000000)), best human Jeopardy (0.705112 (positive:0.733993)), candidate answer (0.697250 (positive:0.646468)), final ranked order (0.695159 (neutral:0.000000)), accomplished human contestants (0.694224 (positive:0.311081)), Watson Pattern-Matches (0.657408 (neutral:0.000000)), single clue (0.641834 (neutral:0.000000)), astonishing thoroughness (0.638067 (positive:0.738805)), massive amounts (0.634453 (negative:-0.383117)), answer-scoring analytics (0.626945 (positive:0.291679)), deep analytics (0.625377 (positive:0.345905)), pattern matching (0.625361 (negative:-0.383117)), newspaper stories (0.622731 (neutral:0.000000)), complex communication (0.621099 (neutral:0.000000)), reference works (0.619658 (neutral:0.000000)), new computer overlords (0.618870 (positive:0.505351)), machine-learning framework (0.618816 (neutral:0.000000)), search results (0.614886 (neutral:0.000000)), search result (0.612805 (neutral:0.000000)), additional searches (0.611919 (neutral:0.000000)), Ken Jennings (0.607432 (neutral:0.000000)), televised tournament (0.607301 (positive:0.311081)), final confidence (0.606248 (positive:0.562324)), digital technologies (0.605667 (negative:-0.422470)), evidence (0.583385 (positive:0.496186))
Entities:
Watson:Person (0.884494 (positive:0.222256)), IBM:Company (0.499916 (neutral:0.000000)), Ken Jennings:Person (0.451165 (neutral:0.000000)), Bible:Organization (0.354451 (positive:0.290197)), research manager:JobTitle (0.330224 (neutral:0.000000)), Eric Brown:Person (0.314400 (neutral:0.000000)), three days:Quantity (0.314400 (neutral:0.000000))
Concepts:
Jeopardy! (0.934769): website | dbpedia | freebase | yago
Ken Jennings (0.870267): website | dbpedia | freebase | yago
Competition (0.821958): dbpedia | freebase | opencyc
Question (0.734962): dbpedia | freebase
Answer (0.734098): dbpedia | freebase
Search engine optimization (0.680755): dbpedia | freebase
Victor J. Kemper (0.666356): dbpedia | freebase | yago
Jeopardy! Ultimate Tournament of Champions (0.620353): website | dbpedia | freebase | yago
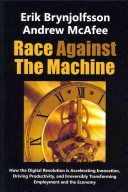
